Poisoned Data in AI Training Opens Back Doors to System Manipulation
Data poisoning presents a grave cybersecurity threat, with malicious actors compromising AI training datasets to manipulate system behavior. As AI becomes integral across key sectors, these attacks highlight urgent gaps in security measures. A Nisos report reveals sophisticated techniques being used and stresses the need for advanced defenses, highlighting the broader implications for sectors like healthcare, finance, and national security.
Essential Designs Team
|
November 16, 2024
.jpg)
Data poisoning presents a growing challenge in cybersecurity, where malicious actors intentionally introduce deceitful or harmful data into AI training datasets. The aim is to sabotage AI systems by skewing outcomes, fostering biases, or even creating vulnerabilities for further exploitation. As artificial intelligence becomes ingrained in essential sectors and everyday life, these attacks raise significant concerns for developers and businesses relying on AI technologies.
The landscape of AI security is in constant flux, with new threats emerging as quickly as innovative defense solutions are developed. A recent study by intelligence firm Nisos highlighted the diverse tactics employed in data poisoning, ranging from simple data mislabeling and injections to more advanced techniques like split-view poisoning and backdoor meddling. This report underscores an alarming trend: the growing sophistication of cyber adversaries, who now employ more precise and difficult-to-detect strategies.
Nisos senior intelligence analyst Patrick Laughlin reveals that even minimal data poisoning, affecting just 0.001% of a training set, can substantially alter AI model performance. This threat has dire implications for industries like healthcare, finance, and national security. "It highlights a pressing need for robust technical measures, organizational protocols, and persistent vigilance to counter these threats effectively," Laughlin shared with TechNewsWorld.
Assessing Existing AI Security Measures
Laughlin pointed out that current cybersecurity measures fall short of addressing these escalating threats. While foundational, they demand enhancement through new strategies tailored to combat evolving data poisoning efforts. The report advocates for AI-enhanced threat detection, the development of inherently resilient learning algorithms, and advanced solutions such as blockchain for maintaining data integrity.
Critical too, are privacy-preserving machine learning techniques and adaptable defense mechanisms that evolve with emerging attack methods. Importantly, these issues transcend business interests, presenting a broader risk to essential domains like healthcare, autonomous transport, and national security infrastructures.
Data poisoning doesn’t just jeopardize system functionality; it also poses a risk to public trust in AI technologies, potentially worsening societal issues such as misinformation and bias proliferation.
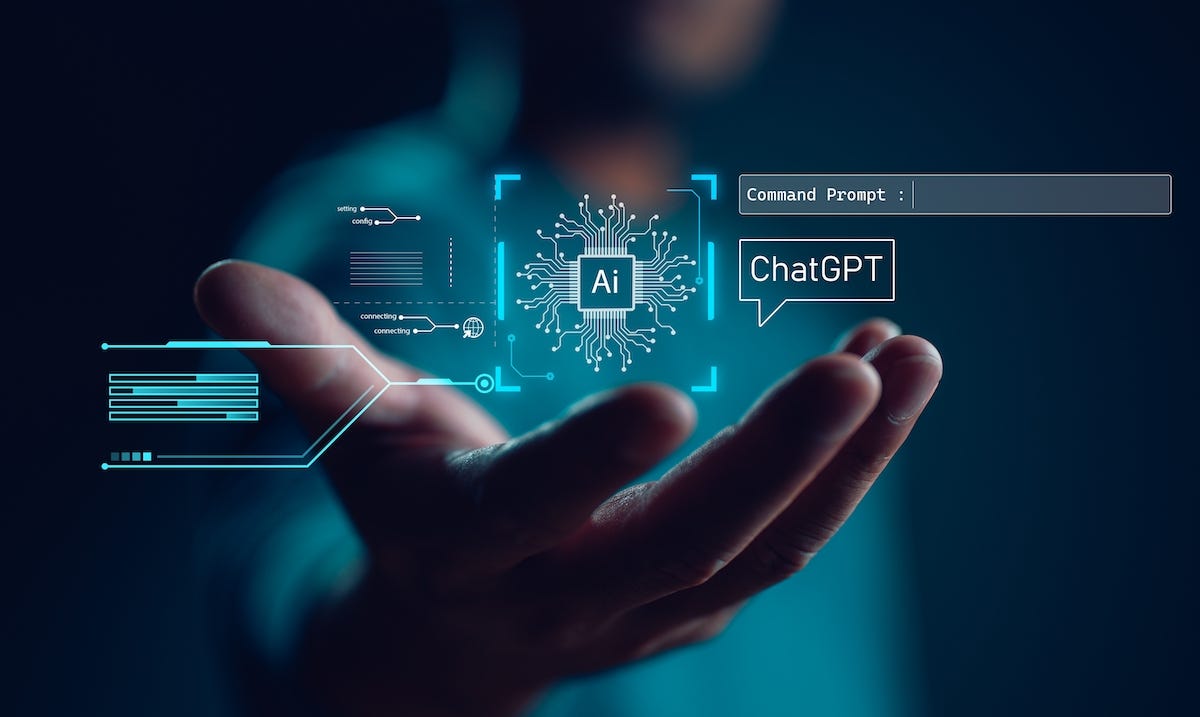
Far-Reaching Impacts
Laughlin cautions that compromised decision-making in pivotal systems represents one of the gravest dangers of such attacks, particularly in scenarios like healthcare diagnostics or the operation of autonomous vehicles, where human lives could be at risk. Financial repercussions and market disruptions are also concerns, as compromised AI systems in the financial sector can lead to significant monetary losses and instability.
National security vulnerabilities may include critical infrastructure weaknesses and the facilitation of widespread misinformation campaigns. Recurring examples illustrate these dangers, such as the 2016 breaches involving Google’s Gmail spam filter and Microsoft's Tay chatbot, both heavily impacted by malicious training data.
Mitigation Strategies
To counteract data poisoning, the Nisos report proposes several strategies. Key among them is establishing rigorous data validation and sanitization processes, alongside continuous AI system monitoring and auditing. The use of adversarial sample training to bolster model robustness, diversifying data sources, ensuring secure data management, and investing in educational initiatives for users are also recommended defenses.
Laughlin advises AI developers to closely manage dataset sourcing, focus on programmatic defenses, and invest in AI-powered threat detection.
The Road Ahead
Looking toward the future, the report warns of fast-evolving data poisoning tactics. Cyber adversaries are adept at learning and innovating, expected to develop more nuanced and evasive techniques. This heightens the vulnerabilities related to emerging AI paradigms like transfer and federated learning systems, which could introduce new avenues for attack.
Balancing AI security with priorities such as privacy and fairness becomes increasingly complex as AI systems grow in sophistication. The call for standardization and regulatory frameworks in addressing AI security comprehensively becomes more urgent.